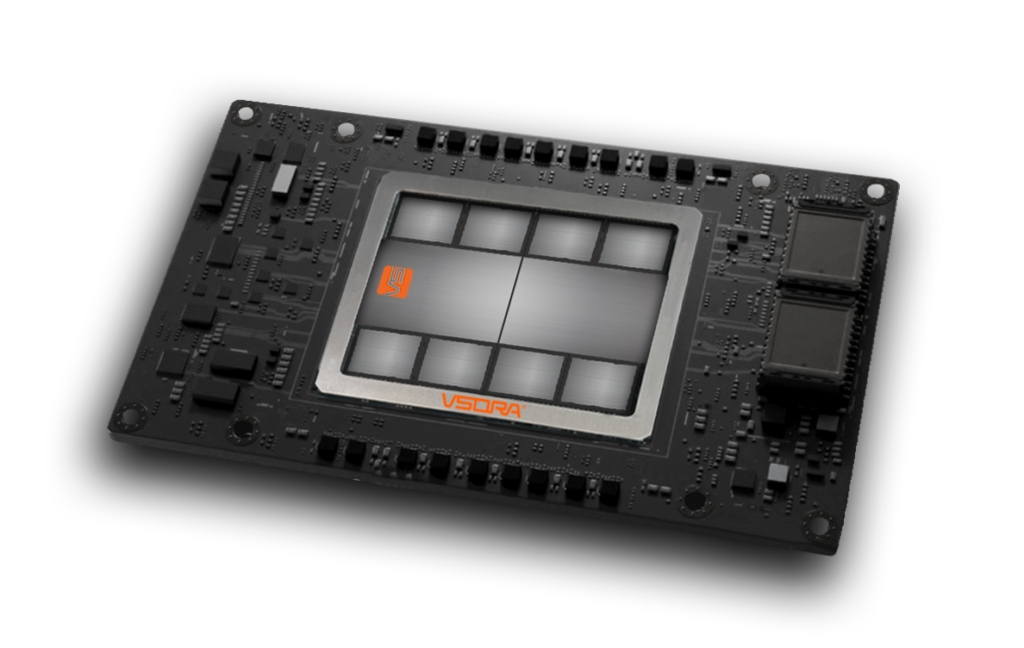
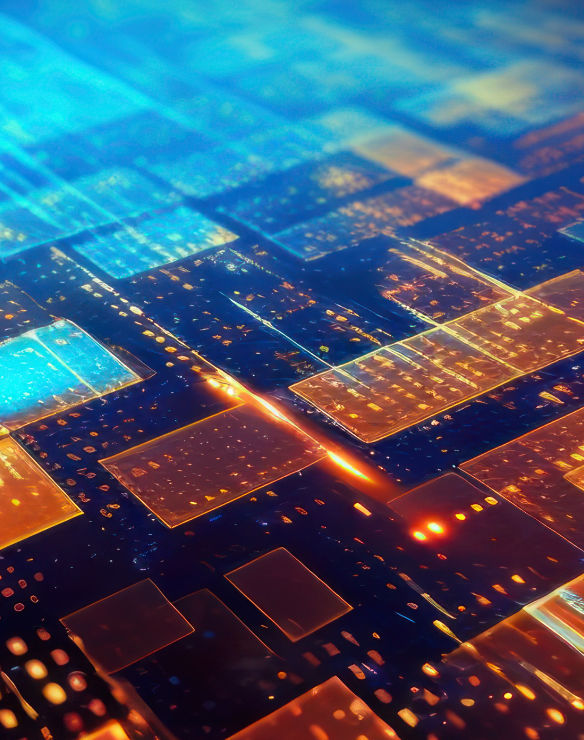
THE ”MEMORY WALL”
Why Generative AI is Ready – But Hardware Isn’t
The Memory Wall, first theorized in 1994, describes how CPU advancements outpace memory speed, causing delays as processors wait for data. Traditional architectures mitigate this with hierarchical memory structures, but Generative AI models like GPT-4, requiring nearly 2 trillion parameters, push these limits.
Current hardware struggles to handle such massive data loads efficiently. Running GPT-4, for example, results in just 3% efficiency, with 97% of computing time spent on data preparation. This inefficiency demands enormous hardware investments—Inflection’s supercomputer, for instance, requires 22,000 Nvidia H100 GPUs, consuming 11 MWh of power.
Jotunn introduces a new architecture that eliminates bottlenecks, ensuring data is continuously fed to processing units. This breakthrough boosts efficiency beyond 50%, making it vastly superior to current solutions.
Our AD/ADAS Offer
Tyr4
3.2 Petaflops for any AD / ADAS application. All completely CUDA-free.
Tyr2
1.6 Petaflops for any AD/ADAS application. All completely CUDA-free.
Tyr1
800 Teraflops for any AD / ADAS application. All completely CUDA-free.
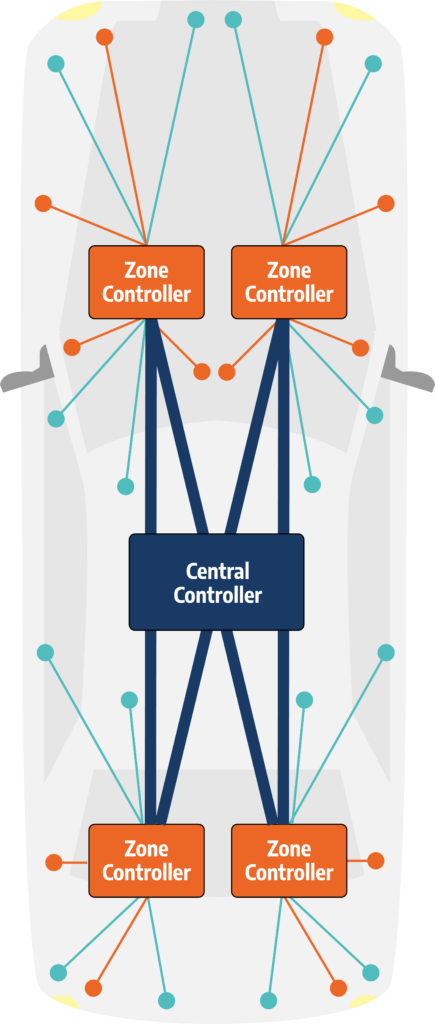