Jotunn8
Large LLM deployment
cost reduced to
2%
of leading solutions
Ex: GPT-4 Cost/Query: <$0.002
Our Generative AI Offer
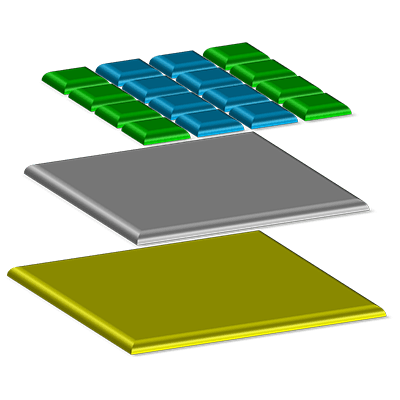
Jotunn8
6.4 Petaflops tailormade for easy, CUDA-free Generative AI use. Large LLMs, like GPT-4, can quickly be implemented and deployed using standard software.
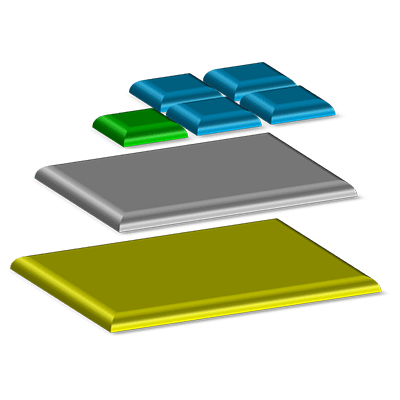
Tyr4
3.2 Petaflops for any AI application, including advanced Generative AI completely CUDA-free
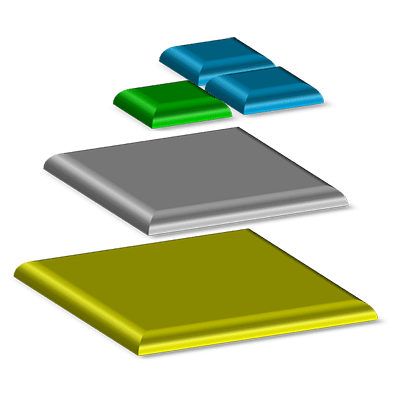
Tyr2
1.6 Petaflops for easy, CUDA-free AI, including Generative AI. All completely CUDA-free
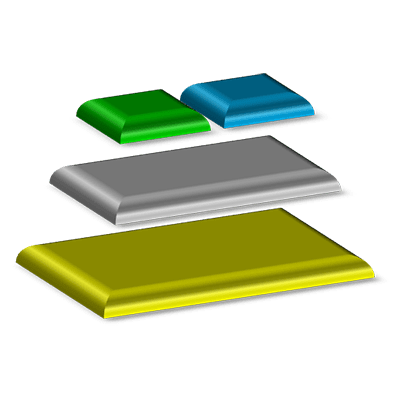
Tyr1
800 Teraflops for any AI application, including Generative AI. All completely CUDA-free
Autonomous Driving / ADAS
CUDA-Free High-Performance
Low-Power Computing
for the Vehicle
3.2 PetaFlops / 60 W
Any Algorithm
Any Host Processor
Fully Programmable
Our AD/ADAS Offer
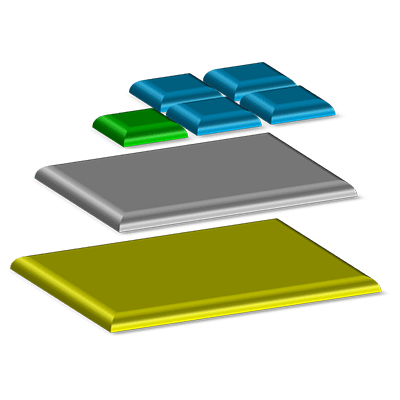
Tyr4
3.2 Petaflops for any AD / ADAS application. All completely CUDA-free.
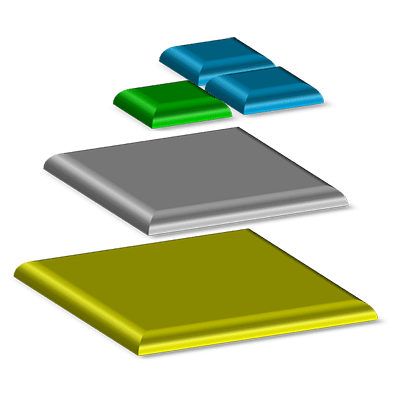
Tyr2
1.6 Petaflops for any AD/ADAS application. All completely CUDA-free.
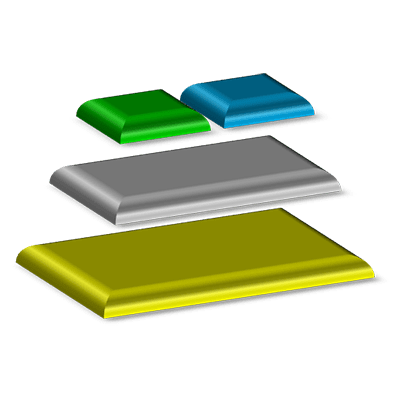
Tyr1
800 Teraflops for any AD / ADAS application. All completely CUDA-free.
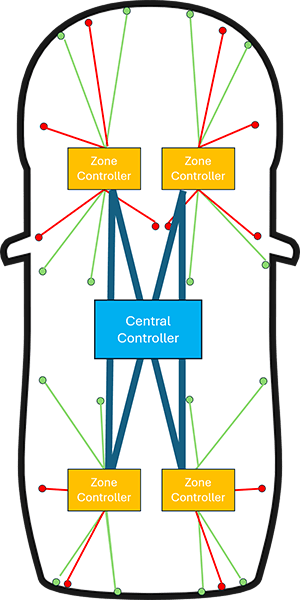
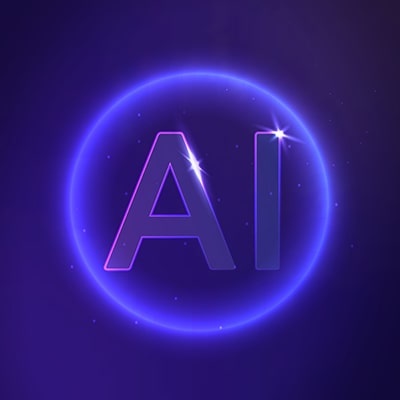
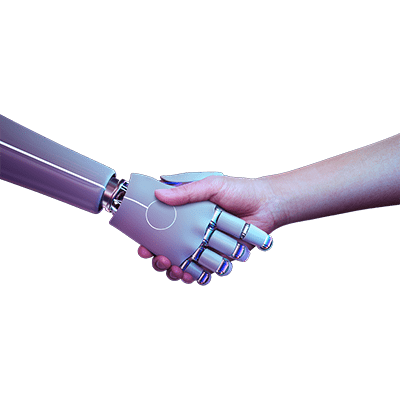